Augmented SBERT
Motivation
Bi-encoders (a.k.a. sentence embeddings models) require substantial training data and fine-tuning over the target task to achieve competitive performances. However, in many scenarios, there is only little training data available.
To solve this practical issue, we release an effective data-augmentation strategy known as Augmented SBERT where we utilize a high performing and slow cross-encoder (BERT) to label a larger set of input pairs to augment the training data for the bi-encoder (SBERT).
For more details, refer to our publication - Augmented SBERT: Data Augmentation Method for Improving Bi-Encoders for Pairwise Sentence Scoring Tasks which is a joint effort by Nandan Thakur, Nils Reimers and Johannes Daxenberger of UKP Lab, TU Darmstadt.
Chien Vu also wrote a nice blog article on this technique: Advance BERT model via transferring knowledge from Cross-Encoders to Bi-Encoders
Extend to your own datasets
Scenario 1: Limited or small annotated datasets (few labeled sentence-pairs (1k-3k))
If you have specialized datasets in your company or research which are small-sized or contain labeled few sentence-pairs. You can extend the idea of Augmented SBERT (in-domain) strategy by training a cross-encoder over your small gold dataset and use BM25 sampling to generate combinations not seen earlier. Use the cross-encoder to label these unlabeled pairs to create the silver dataset. Finally train a bi-encoder (i.e. SBERT) over your extended dataset (gold+silver) dataset as shown in train_sts_indomain_bm25.py.
Scenario 2: No annotated datasets (Only unlabeled sentence-pairs)
If you have specialized datasets in your company or research which only contain unlabeled sentence-pairs. You can extend the idea of Augmented SBERT (domain-transfer) strategy by training a cross-encoder over a source dataset which is annotated (for eg. QQP). Use this cross-encoder to label your specialised unlabeled dataset i.e. target dataset. Finally train a bi-encoder i.e. SBERT over your labeled target dataset as shown in train_sts_qqp_crossdomain.py.
Methodology
There are two major scenarios for the Augmented SBERT approach for pairwise-sentence regression or classification tasks.
Scenario 1: Limited or small annotated datasets (few labeled sentence-pairs)
We apply the Augmented SBERT (In-domain) strategy, it involves three steps -
Step 1: Train a cross-encoder (BERT) over the small (gold or annotated) dataset
Step 2.1: Create pairs by recombination and reduce the pairs via BM25 or semantic search
Step 2.2: Weakly label new pairs with cross-encoder (BERT). These are silver pairs or (silver) dataset
Step 3: Finally, train a bi-encoder (SBERT) on the extended (gold + silver) training dataset
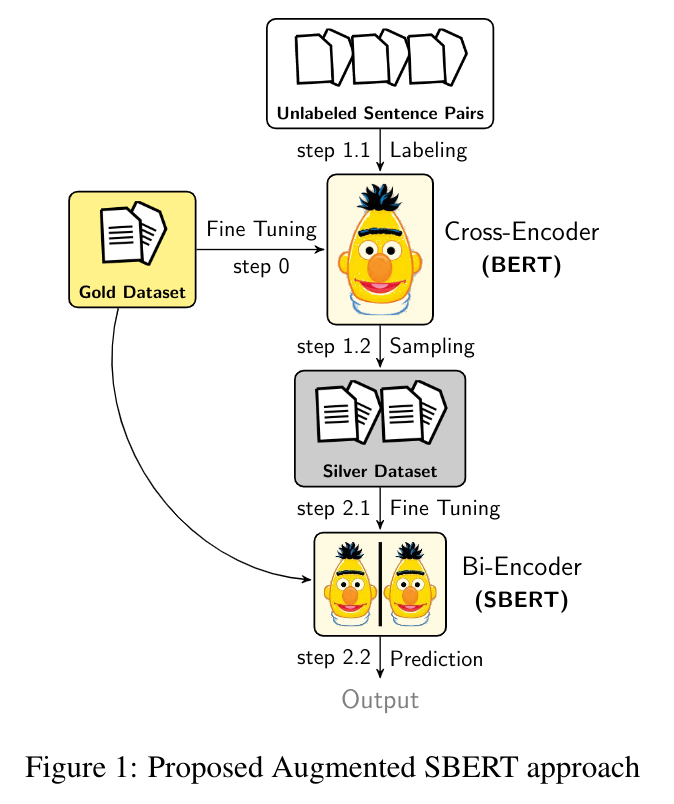
Scenario 2: No annotated datasets (Only unlabeled sentence-pairs)
We apply the Augmented SBERT (Domain-Transfer) strategy, it involves three steps -
Step 1: Train from scratch a cross-encoder (BERT) over a source dataset, for which we contain annotations
Step 2: Use this cross-encoder (BERT) to label your target dataset i.e. unlabeled sentence pairs
Step 3: Finally, train a bi-encoder (SBERT) on the labeled target dataset
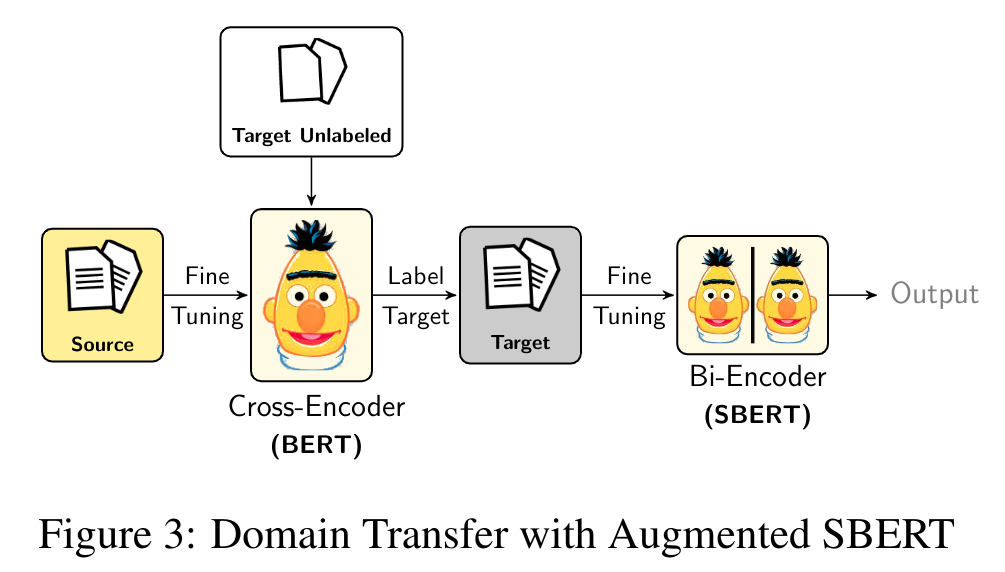
Training
The examples/training/data_augmentation folder contains simple training examples for each scenario explained below:
train_sts_seed_optimization.py
This script trains a bi-encoder (SBERT) model from scratch for STS benchmark dataset with seed-optimization.
Seed optimization technique is inspired from (Dodge et al., 2020).
For Seed opt., we train our bi-encoder for various seeds and evaluate using an early stopping algorithm.
Finally, measure dev performance across the seeds to get the highest performing seeds.
-
This script trains a bi-encoder (SBERT) model from scratch for STS benchmark dataset using easy data augmentation.
Data augmentation strategies are used from popular nlpaug package.
Augment single sentences with synonyms using (word2vec, BERT or WordNet). Forms our silver dataset.
Train bi-encoder model on both original small training dataset and synonym based silver dataset.
-
Script initially trains a cross-encoder (BERT) model from scratch for small STS benchmark dataset.
Recombine sentences from our small training dataset and form lots of sentence-pairs.
Limit number of combinations with BM25 sampling using Elasticsearch.
Retrieve top-k sentences given a sentence and label these pairs using the cross-encoder (silver dataset).
Train a bi-encoder (SBERT) model on both gold + silver STSb dataset. (Augmented SBERT (In-domain) Strategy).
train_sts_indomain_semantic.py
This script initially trains a cross-encoder (BERT) model from scratch for small STS benchmark dataset.
We recombine sentences from our small training dataset and form lots of sentence-pairs.
Limit number of combinations with Semantic Search sampling using pretrained SBERT model.
Retrieve top-k sentences given a sentence and label these pairs using the cross-encoder (silver dataset).
Train a bi-encoder (SBERT) model on both gold + silver STSb dataset. (Augmented SBERT (In-domain) Strategy).
-
This script initially trains a cross-encoder (BERT) model from scratch for STS benchmark dataset.
Label the Quora Questions Pair (QQP) training dataset (Assume no labels present) using the cross-encoder.
Train a bi-encoder (SBERT) model on the QQP dataset. (Augmented SBERT (Domain-Transfer) Strategy).
Citation
If you use the code for augmented sbert, feel free to cite our publication Augmented SBERT: Data Augmentation Method for Improving Bi-Encoders for Pairwise Sentence Scoring Tasks:
@article{thakur-2020-AugSBERT,
title = "Augmented SBERT: Data Augmentation Method for Improving Bi-Encoders for Pairwise Sentence Scoring Tasks",
author = "Thakur, Nandan and Reimers, Nils and Daxenberger, Johannes and Gurevych, Iryna",
journal= "arXiv preprint arXiv:2010.08240",
month = "10",
year = "2020",
url = "https://arxiv.org/abs/2010.08240",
}